GDMSS Lite PC Windows 7 – How to download gDMSS Lite for Windows 10 Laptop. GDMSS Lite Apk is free to download for Android devices and in the same way you can use that to download gDMSS Lite for Windows 10 PC or the previous Windows 8.1/8/7/XP PC.
« Previous: Chapter 8 - Open-Ended Survey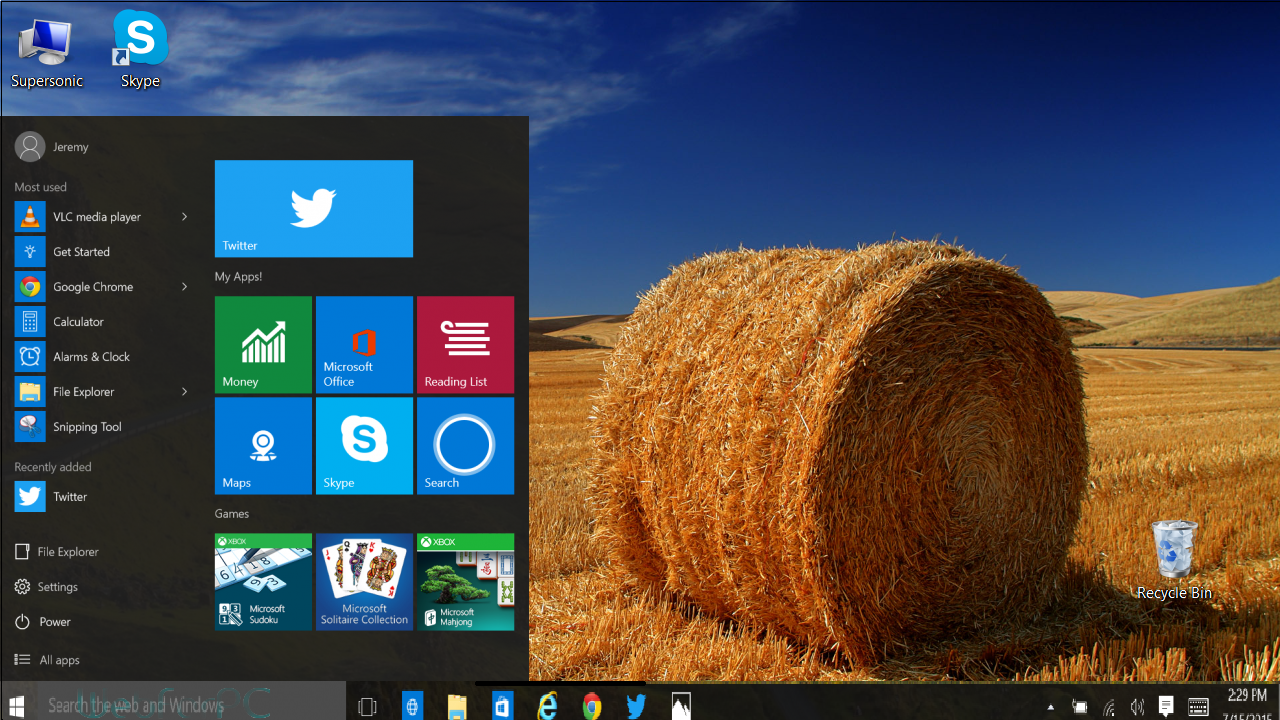
Below is the uncorrected machine-read text of this chapter, intended to provide our own search engines and external engines with highly rich, chapter-representative searchable text of each book. Because it is UNCORRECTED material, please consider the following text as a useful but insufficient proxy for the authoritative book pages.
85C h a p t e r 9Utility is a measure of absolute or relative satisfaction. Con-versely, disutility is a measure of dissatisfaction. In the case of travel, disutility assessments translate the dissatisfaction asso-ciated with travel into quantified and monetized measures. Trip disutility is the complex and collective bottom-line out-come that takes into account interactions among traveler needs and preferences, trip purpose, travel conditions, per-ceived and objective trip time, and travel time uncertaintyâin addition to the direct costs of travel, such as fuel or fares. Trip disutility functions assign value, or cost per unit, for spe-cific components associated with trips, such as travel time and schedule delay. Valuations based on reductions in travel disutility are central to cost-benefit analyses for transporta-tion investments and, more recently, for traffic operations decisions such as value pricing of high-occupancy toll lanes.Many studies have assessed the value of travel time, sched-ule delay, and reliability associated with travel; and a number have further refined the valuation to reflect specific traveler and modal characteristics. Fewer studies further assess reduc-tions in trip disutility resulting from the provision of traveler information. What is still relatively unexplored is the mone-tized reduction in trip disutility associated with the provision of travel time reliability information. Travel time reliability information, as defined in the SHRP 2 L14 effort, encom-passes a broad range of information that describes underlying trip time variability and other contextual data travelers use to manage delay and on-time performance. The information encompasses three general categories: (a) descriptions of the statistical variation in travel time dependent on departure time choice, (b) data regarding on-time performance and late-ness risk by route and destination, and (c) contextual informa-tion to interpret cueing throughout the travel experience to allow travelers to better assess travel time and lateness risk both pre-trip and en route. Travelers who make repeated simi-lar trips at the same time of day accumulate an internalized assessment of trip travel time variability with which to gauge lateness risk. Relative to those familiar trips, researchers expect thatâfor unfamiliar tripsâtravelers will perceive a larger benefit from travel time reliability information.The travel behavior laboratory experiment described in this chapter aimed to measure the perceived value of trip reliability information and how that valuation changes with increasing trip familiarity. This assessment of how reliability information can reduce travel disutility included the consideration of a particular aspect, trip serenity, or the reduction of stress en route associated with potential late arrival. The experiment captured variations in reported stress associated with on-time performance at trip start, while en route, and at trip end among scenarios with varying levels of traveler information and trip outcomes.The objectives of the experiment were twofold: (a) to assess the value participants placed on traveler information, specifi-cally reliability information, within the context of a simulated time-constrained trip; and (b) to determine whether having reliability information helped speed the transition from an unfamiliar commuter to an experienced commuter. The under-lying conjecture in the experiment was that providing reliabil-ity information would allow an unfamiliar traveler to manage the trade-off between on-time performance and travel budget (time allocated to travel) more effectively and with less stress. Put another way, the provision of reliability information to travelers unfamiliar with underlying travel time variability patterns was expected to both improve overall trip outcomes (reduction of early and late schedule delay, better on-time performance, and reduced delay) and reduce perceptional disutility associated with those improved outcomes. Three experimental hypotheses were posed:1. Provision of accurate reliability information will result in improved on-time performance and lower generalized travel disutility compared with a control group receiving no reliability information.2. While travel outcomes improve with the provision of reli-ability information, the perceived value of the reliability Travel Behavior Laboratory Experiment
86experiment was also designed to work within the implemen-tation framework of the existing SHRP 2 L14 computer survey task. The experiment was developed by Noblis and deployed by TTI in concert with the planned computer surveys.Literature reviewThe valuation of individualâs travel has been of interest in transportation research for decades, with earliest valuations focusing on the travel time component of travel. Valuation of travel time for work trips expanded to valuation by travel mode and to nonwork trips. Since the 1960s, studies through econo-metric, stated preference, and revealed preference models have assessed valuation of travel. Over time, researchers have devel-oped more complex frameworks for this valuation to more truly reflect actual valuation by travelers by considering factors such as travel conditions (e.g., congestion, reliability) and trav-eler characteristics (e.g., trip purpose, scheduling flexibility).Vickery (1963) suggested expanding travel valuation beyond travel time to consider the concept that, for the work com-mute, the penalty for being early would be less than for being late. The concept of time constraints on a trip and travelersâ preference for a specific arrival time was furthered by Gaver (1968), who hypothesized that trip disutility is a linear func-tion of travel time and early and late schedule delays. Small (1982) built on earlier work using a scheduling delay frame-work to explicitly tie departure time choice to the utility func-tion of a traveler. Using data from 527 San Francisco Bay Area auto commuters, Small formulated a model with specific components for travel time, early arrival, late arrival, and a fixed late-arrival penalty. Hendrickson and Plank (1984), using journey-to-work data from Pittsburgh, deconstructed the valuation to differentiate free-flow travel time, congested travel time, modal costs, linear early and late arrival, and qua-dratic terms for early and late arrival. Their work confirmed that, for the work trip, late arrival has far greater disutility compared with early arrival and that, once an individual is quite late for work, additional late time has relatively little additional disutility.A parallel theoretical framework was proposed by Jackson and Jucker (1982), in which trip disutility is a function of the expected travel time and travel time variance; the latter reflects the valuation of reliability but without differentiation for trip arrival outcome. Polak (1987) proposed alternatives in which the variability term can be quadratic or exponential (for travelers with absolute risk aversion). Black and Towriss (1993) then extended this work to develop a reliability ratio as the ratio of the value of reliability to the value of time.Noland and Small (1995) combined those two approaches by explicitly including the uncertainty of travel time in the trip scheduling approach through a random variable that reflects the travel time variability. Small et al. (1999) conducted surveys information will underestimate the realized benefit in terms of reduced delay, improved on-time reliability, and reduced stress.3. The benefits of reliability information will decline over time as both experimental and control subjects learn and understand the underlying travel time variability. That is, the benefit from reliability information during the first weeks will be larger than during the last weeks.To meet experimental objectives and to best fit within the overall SHRP 2 L14 project, Noblis selected a trip simulation approach to the experiment. The experimental strategy and corresponding evaluation tools were prepared by Noblis, while the experiment was administered by the TTI staff. Data gener-ated by the experiment were analyzed by Noblis in support of the SHRP 2 L14 Task 12, Development of Utility Functions.The experiment presented 80 participants with a scenario in which they were commuting in an unfamiliar city with a constrained arrival time (with penalties for both late and early arrival) for two work weeks (10 commutes). Participants had access to one of three additive levels of travel time informa-tion for their planned route and optional route deviations: real-time advisory messages only, real-time advisories plus real-time travel time information, or real-time advisories plus both real-time and reliability (historical travel time range) information. Participants selected from among three depar-ture time options and two potential alternate route options to arrive at their destination on time. All participants received the real-time advisory messages (from simulated DMSs deployed along the simulated route); a subset of participants had to actively acquire the higher levels of real-time and reliability information by pressing an interface button. The experiment was conducted in the same five cities (Dallas, Denver, Hartford, Miami, and San Jose) as the SHRP 2 L14 computer survey.BackgroundWithin the SHRP 2 L14 project, two activitiesâa driver sim-ulation study and a field testâwere expected to generate data that would be leveraged to measure the perceived value of trip reliability informationâthe goal of Task 12, Development of Utility Functions. However, the elimination of the simulator testing along with a recasting of planned field testing pre-cluded the acquisition of data critical to the completion of Task 12. At the same time, findings from Phase 1 focused attention on quantifying the relative value of reliability infor-mation. Because of these changes in project direction, Noblis and TTI proposed an experiment using subjects traversing a simulated trip to meet the goals of Task 12. The experiment was designed to assess the serenity benefits from trip reliabil-ity information and to translate the value of trip reliability information through assessment of trip disutility levels. The
87 were given information (static value of travel time) for mul-tiple routes. Travelers were asked to make a trip using multi-ple route options. Participant travel was recorded through GPS units, and participants were then asked to rank order travel among the multiple routes as well as provide ratings on the quality of the trip. Using regression, multinomial, and rank-ordered models, the authors founds that travelers had perception biases and systematically misperceived certain aspects of their trip. Furthermore, accurate information was valued only for commute and event trips, and not for other trip purposes, such as recreation.Basu and Maitra (2010) applied a stated preference approach to compare traveler (both taxi and private vehicle) valuation of traffic information on a variable message sign. Informa-tion was presented in two forms: instantaneous travel time and an additive level of information, which was a categorical variable for variation in instantaneous travel time from the previous estimate. The application of two different types of logit models resulted in valuation of travel time variation at par or greater than the value of travel time for private-car trip makers. For taxi trip makers, both models indicated that valuation of travel time variation was greater than travel time.experiment planSeveral iterations of an initial experimental design were con-sidered by the Noblis team. Constraints of overall testing ses-sion length and resources required for participant sample size restricted the number of factorial combinations that could be tested. The final chosen form focused specifically on making an initially unfamiliar, routine, time-constrained trip. The travel behavior experiment was programmed using Visual Basic for Applications (VBA) within the Microsoft Excel envi-ronment. Several iterations of quality assurance and testing were completed on the multiple laptop computers used in the field to create accurate visual appearance and macro execu-tion given the varying performance levels and settings among field laptops.The experiment was conducted in five citiesâDallas, Denver, Hartford, Miami, and San Joseâover a period of 4 months and acquired information from 80 participants. Experiment participants were recruited through advertise-ments on craigslist.org. Table 9.1 presents the number of participants by city. The cities were selected to include a diverse survey population that had experience with varying levels of traffic congestion, commute lengths, and highway network connectivity.Figure 9.1 presents the screenshots of the Excel-based interface that served as the starting point for experiment participants, as well as a sample experiment screenshot. Each participant was seated in front of a dedicated laptop with a mouse and was required to maneuver the mouse to click of thousands of households in southern California and found that the value individuals place on travel time and trip reli-ability varies depending on the level of congestion. Further-more, on the basis of this large stated preference survey, researchers found that a quadratic term for early schedule delay best reflects observations. Below are the form and esti-mated parameters of the 1999 research by Small et al. Both were applied to the current experiment to compare perceived benefit measures with utility-based outcomes.2 22c T SDE SDE SDL DSDE SDE L( ) ( ) ( )= α +β + β + γ +θwhere c = cost of travel (disutility) T = travel time SDE = schedule delay early SDL = schedule delay late DL = late-arrival index = 1 if 00 otherwiseSDL >The estimates of the parameters are a = $0.0564/min (linear cost of in-vehicle travel time) bSDE = -$0.023/min (linear component of quadratic early cost) bSDE2 = $0.005/min (quadratic component of quadratic early cost) g = $0.310/min (linear cost of late arrival) q = $2.87 (one-step penalty for arriving late)Small et al. (2005) found that commuters differ substantially in how they value travel time and reliability, and that the aver-age valuation of both is quite high and is considerably higher when measured in real as opposed to hypothetical scenarios. Recent work has explored variants in the form of the early- and late-arrival components as well as the form by which to repre-sent travel time variance (e.g., Bates et al. 2001; Tilahun and Levinson 2010). Other research addressed the inclusion of risk attitudes in trip scheduling models (Senbil and Kitamura 2004; Li 2010) and extended the formulation to consider trip chains (Jenelius et al. 2011).With the growth in public real-time traveler information in the 1990s, travel valuation research expanded to valuation of traveler information, as it not only provides trip makers the ability to save travel time but also provides serenity ben-efits and the ability to shift activities to accommodate trip uncertainty. Many studies have estimated the system-level travel time savings from traveler information through network simulation models (e.g., Wunderlich et al. 1999), while others have focused on willingness to pay through stated preference surveys (e.g., Khattak et al. 2003).Zhang and Levinson (2006) focused on the benefits of reductions in driver uncertainty when traveler information is provided at the beginning of a trip. In that study, travelers
88information framed the characteristics of participants and can be compared against the population at large or with other future experimental groups. The travel purpose, schedule integrity, and roadway usage questions together identified the types of trips for which on-time arrival was most important to participants, the frequency with which those trips were made, and whether the trips for which on-time arrival was most critical were made on roadways where traveler infor-mation is usually available. The detailed descriptions and outcomes of the pre-experiment survey are presented later in this chapter.The moderator began the commute experiment with instruc-tions to the participant group. The moderator read aloud the contextual setup text presented in the experiment and informed participants that different individuals would have on interface buttons, radio buttons, or drop-down menus. Participants did not use the keyboard during the experiment. On arrival at the survey room, participants were seated and provided a brief explanation about the intent of the experi-ment. Participants completed a pre-experiment survey of 10 complex questions, followed by a group-led experiment and a post-experiment four-question survey. Table 9.2 sum-marizes the information requested in the three sections of the experiment.Participants independently completed the pre-experiment survey (âBegin Surveyâ button in Figure 9.1) and waited for a moderator to indicate the start of the commute experiment (âBegin Experimentâ button in Figure 9.1). The pre-experiment survey requested socioeconomic, travel purpose, roadway usage, and schedule integrity questions. The socioeconomic Table 9.1. Responses in Commute Experiment, by City and Type of Information ReceivedCity Experiment DatesParticipant Count VMS VMS 1 RTVMS 1 RT 1 HISTORICDallas 2/14/2011, 2/15/2011 15 6 5 4Denver 12/8/2010, 12/9/2010 15 3 4 8Hartford 1/18/2011â1/20/2011 21 9 7 5Miami 11/30/2010â12/2/2010 10 1 3 6San Jose 1/11/2011â1/13/2011 19 7 5 7Total 80 26 24 30Note: VMS = variable message sign (also known as changeable or dynamic message sign, CMS or DMS), RT = real-time travel time, HiSToRiC = reliability or historical travel time range information.Figure 9.1. Interface view when participants were seated for the experiment.
89 Pre- and Post-Experiment SurveysThe pre-experiment and post-experiment surveys developed a baseline of participant characteristics that were useful in comparing participant characteristics with the population at large. Key data acquired through the surveys included gender, age, educational level, and traveler information usage. Addi-tionally, the survey questions aimed to explore whether the participant population made trips that would benefit most from traveler informationâthat is, trips that were sufficiently long, required stringent on-time arrivals, and used facilities for which such information would be available (i.e., high-ways). Participants with these routine trip-making character-istics were likely to better internalize trade-offs requested in the simulation experiment, and their responses were more likely to reflect true trip decision making. The specific text of pre- and post-experiment questions and the response options for questions are presented in Appendix F.The survey questions also enabled researchers to develop lateness thresholds, that is, to explore what participants per-ceived as arriving late according to their specification for on-time arrival importance and trip purpose. The thresh-olds can be applied in future work to refine the form of utility functions.Willingness to pay for guaranteed travel time (zero trip variability or 100% trip reliability) and willingness to pay for knowledge of late arrival were both assessed to compare with sensitivities observed through the experiment. A priori, par-ticipants with high on-time arrival importance were expected to be willing to pay more for this guarantee or arrival out-come information.At the end of the simulation experiment, the survey asked participants what their most important objective was when making departure time decisions (calling family, reducing access to differing levels of traveler information. Participants were instructed not to share their successful or failed com-mute strategies with each other to prevent participants with lower levels of traveler information from acquiring insight afforded to participants with more precise traveler informa-tion. The moderator supported participants for the first day of travel by presenting on large visual images the location of specific types of information and decision sets. Thereafter, participants used experiment information and made com-mute decisions at their own pace to complete commutes to work. Although the intent of the experiment was to have par-ticipants complete 20 commute days (1 commute month), time and resource constraints required curtailing the experi-ment to 10 commute days.Participants indicated willingness to pay for traveler infor-mation, on-time arrival confidence level, trip stress level, sat-isfaction level with trip outcome, and traveler information usefulness for each of the 10 completed trips. Data support-ing the experiment were designed to represent a moderate level of trip variability as well as temporal consistency among departure time, traveler information, and trip experiences. The details related to the simulation data set along with the outcomes from the commute experiment are presented later in this chapter.As with the pre-experiment survey, the moderator instructed participants to complete the post-experiment survey inde-pendently. The post-experiment survey was brief, consisting of four questions. This survey asked participants to rate the usefulness of traveler information at the beginning and toward the end of the experiment. That information was used to cor-roborate participantsâ responses within the commute experi-ment. The format and findings of the post-experiment survey are presented later in this chapter.Table 9.2. Information Acquired from ExperimentPre-Survey Information Acquired Experiment Information Acquired Post-Survey Information Acquired⢠Gender, age, education⢠Travel frequency by trip purpose (work, educa-tion, childcare, medical, recurring social)By Trip Purpose for trips with frequency selection other than ânoneâ:⢠on-time arrival importance⢠Average travel time⢠âBad dayâ additional time Quantification of âlateâ⢠Willingness to pay travel time guarantee⢠Willingness to pay knowledge of late impending arrival en-route time guaranteeParticipants selected the following for the begin-ning and subsequent segments of each trip (as applicable):⢠whether to view traffic information⢠willingness to pay amount for information viewed⢠start time for trip⢠route selection⢠level of confidence for an on-time arrival⢠level of stress for the trip thus farAt the end of each trip, participants also selected the following:⢠overall level of stress for the entire trip⢠level of happiness with trip outcome⢠overall level of usefulness of traffic information⢠willingness to pay amount for all the information viewed during this trip.⢠Usefulness of traffic info at�â beginning of experiment�â end of experiment⢠Sources of traffic information participant uses for their travel (radio, television, computer, handheld or dashboard devices)
90for a participant with L3 traveler information, after the par-ticipant had clicked on the âtraffic primary routeâ button.A qualitative DMS message was visible at the start of the trip (H in Figure 9.2) and at two waypoints along the trip. The DMS message sets are presented in Appendix F along with their frequency of use. Participants chose route options and whether to view additional travel information, if available to them, at Waypoints 1 and 2. During the commute, participants had information (left side of Figure 9.2) regarding the current simulation time, time remaining for them to complete their trip on time, time to traverse the previous segment of road-way, and when they had started their trip.Specific questions regarding levels of trip stress and on-time arrival confidence had to be completed at each waypoint and at the beginning and end of each trip, as relevant. The specific types of data collected through the simulation experiment are presented in Appendix F.Among the 10 commute days, half were non-event days and half were event days, as listed in Table 9.3. The order of days remained consistent among all participants. Commute times were designed for temporal and route consistency and were reflective of event and non-event days. Table 9.4 and Table 9.5 present average travel times and range of travel time by time of departure and route, for non-event and event days, respec-tively. The specific travel times for each day are presented in Appendix F. Given the simulation travel times, the optimal departure time to meet the schedule-constrained arrival of 9:00 a.m. was 8:15 a.m. for non-event days and 8:00 a.m. on all event days except for day 8, the heavy rain day. On that day, a 7:45 a.m. departure time was required for an on-time arrival.The DMS, real-time, and reliability data that were presented to participants aligned with the commute times they experi-enced. That is, the quality of the traveler information was good. Only on days 5 and 10 did the real-time travel time estimate at the beginning of the trip differ significantly from experience, and that was because accidents were simulated to occur after trip departure.pre- and post-experiment Survey FindingsThe pre- and post-experiment surveys provided insight on the characteristics and preferences of participants. All 80 partici-pants completed the surveys. A greater subset of participants made work and medical trips with some frequency compared with school, child-care, or other routine trips. Work and medi-cal trips more often use highways, are longer than school or child-care trips, and have higher requirements for on-time arrival than child-care or other routine trips. Consequently, trips made to work and medical appointments are ideal for using traveler information. However, familiarity with traffic for routine work trips will likely make reliability information parking fee, avoiding late arrival, arriving exactly on time). The survey also asked how useful they found the traveler informa-tion at the beginning and end of the trip. The data provided a clearer holistic understanding of what drove participantsâ departure choices and whether a value loss occurred in their rating of traveler information usefulness.Experiment DescriptionThe key goals of the experimental design were (a) to assess the value participants placed on traveler informationâand the reliability information componentâwithin the context of a simulated time-constrained trip; and (b) to determine whether having reliability information helped speed the transition from an unfamiliar commuter to an experienced commuter. The expectation was that participants with higher levels of traveler information would assign more positive valuation to ques-tions regarding usefulness of traffic information, trip stress, and trip outcome satisfaction during the first week while they were unfamiliar with the trip variability. Furthermore, the expectation was that those with higher levels of information were likely to perform better on event and non-event days because higher levels of information would speed the transi-tion from unfamiliar to experienced commuter. In making these determinations, researchers conducted comparisons of mean valuation among participants with different levels of information and computation of utility values based on trip outcomes.Appendix F presents the narrative given on screen and com-municated verbally to prepare participants for the simulation experiment. In essence, participants were in a new town for work and had to arrive on time to work or otherwise incur a $25 late-arrival fee. Once during the simulation experiment, participants could inform their employer of a late arrival and avoid the late-arrival fee. Early arrival cost (parking cost) was introduced at $4.00 per hour, and an incentive for later depar-ture was introduced in the form of time spent video-chatting with family back home. Participants were asked to select among three departure times (7:45 a.m., 8:00 a.m., and 8:15 a.m.) and multiple routes for a simulated trip to work over a 2-week period, totaling 10 commutes.Participants received one of three levels of information: L1 participants received only qualitative information pre-trip and en route. L2 participants received the same qualitative information as L2 and also could view a real-time estimate of route-specific travel time to their destination. Real-time infor-mation was presented in the simulation at 5-min increments. L3 participants had access to the same information as L2 and also could view static travel time reliability information.Figure 9.2 presents the trip network and identifies the way-points along the trip where qualitative DMS messages were presented. The image in Figure 9.2 is from the simulation tool
91 Table 9.3. Description of Simulated CommutesSimulation Day Description of Day Ideal Departure Timea Alternate Route Far BetterbWeek 1, Day 1 Non-event traffic day 8:15 a.m. NoWeek 1, Day 2 Higher than usual traffic, moderate delays all routes 8:00 a.m. NoWeek 1, Day 3 Non-event traffic day 8:15 a.m. NoWeek 1, Day 4 Non-event traffic day 8:15 a.m. NoWeek 1, Day 5 Minor accident at 7:50 a.m. affecting all routes, minor delays 8:00 a.m. NoWeek 2, Day 6 Blocked lane on primary route from 7:15 a.m. to 8:00 a.m., moderate delays8:00 a.m. YesWeek 2, Day 7 Non-event traffic day 8:15 a.m. NoWeek 2, Day 8 Heavy rain day, significant delays on all routes 7:45 a.m. NoWeek 2, Day 9 Non-event traffic day 8:15 a.m. NoWeek 2, Day 10 Minor accident at 8:00 a.m. affecting primary route 8:00 a.m. Noa ideal departure time avoids late arrival and minimizes excessive early arrival.b Alternate route is equivalent for most days, superior for one simulated day.Figure 9.2. Road network for trip simulation.
92Participants often used multiple media for acquiring traffic information. Among the 80 participants, nearly 60% used radio, while television, computer, and handheld or dashboard devices were used by 43%, 28%, and 23%, respectively.The expectation was that eliminating trip variability would be valued far more than learning en route that arrival would be delayed. According to survey responses, however, partici-pants were willing to pay only slightly more to eliminate travel time variability than to be forewarned of a late arrival. As expected, more important on-time arrivals translated to a greater willingness to pay. Furthermore, among the various trip purposes, participants were willing to pay most to elimi-nate trip variability for work trips and to know of a late work arrival. On average, across all trips, participants indicated that they would be willing to pay $0.85 per trip to eliminate travel time variability and $0.72 to be informed of a late arrival. Table 9.7 lists participantsâ average willingness to pay by trip purpose and by importance of on-time arrival.Participantsâ valuation (perceived usefulness) of the traffic information solicited through the post-experiment survey was neutral to somewhat useful at the start of the experiment. The neutrality diminished with transference more to the âsomewhat usefulâ category and the ârarely/not usefulâ cate-gories. The relative valuation between the three levels of infor-mation received was only moderately different. Approximately 20% of participants who received DMS-only information (L1) negatively rated its usefulness. Surprisingly, only 8% of L2 participants negatively rated its usefulness, while 13% of L3 participants negatively rated its usefulness. The differences in outcomes, however, were not statistically significant.a lower value add-on compared with real-time information. Conversely, when planning nonroutine work or medical appointment trips, reliability information will provide a higher add-on value compared with only real-time information.What participants meant by late was far more precise (within 2 min of scheduled arrival time) for those making work (41% of participants) and child-care trips (53% of participants); all participants making school trips selected a lateness threshold of 10 min or less. For these participants with a narrow lateness threshold, trip reliability played a significant role in budgeting travel time for their trip. These participants were also willing to pay more for a guarantee of travel time or to know early in a trip that they would arrive late.Table 9.6 lists the average minutes late participants charac-terized as arriving late. Across all participants and trip pur-poses, the average threshold for characterizing a trip as late was 6.7 min beyond the desired arrival time. As expected, individuals who indicated arriving on time was very impor-tant had a far smaller lateness threshold. For example, partici-pants who indicated that an on-time arrival at work was very important (category 5) believed that arriving 4.9 min past their scheduled arrival time was considered late, whereas this value was 17.0 min for participants who indicated that on-time arrival at work was somewhat important.Given that the experiment simulated a work trip, partici-pants were asked to identify how frequently they sought out traffic information for this type of trip. More than 90% of par-ticipants who made work trips also sought out traffic informa-tion once or more per week. Among the participants that made daily work trips, 40% also sought out traffic information daily. Table 9.4. Non-Event Day Travel Time ProfilesDeparture TimeAverage and Range of Travel Time by Route for Non-Event DaysPrimary Option 1 Option 2 Option 1 & 27:15 AM 32.8 (31â35) 36.4 (34â39) 34.2 (33â36) 37.6 (35â41)8:00 AM 37.2 (34â41) 40.4 (38â43) 39.4 (36â43) 42.0 (38â43)8:15 AM 41.2 (38â45) 44.6 (40â47) 43.6 (41â47) 45.6 (44â49)Table 9.5. Event Day Travel Time ProfilesDeparture TimeAverage and Range of Travel Time by Route for Event DaysPrimary Option 1 Option 2 Option 1 & 27:15 AM 47.6 (37â64) 45.8 (37â61) 48 (37â63) 46.6 (38â62)8:00 AM 56.4 (47â66) 53.6 (45â66) 56 (42â68) 52.4 (38â65)8:15 AM 57.8 (47â71) 56.4 (39â71) 57.8 (47â73) 55.6 (42â74)
93 highways. Only 9% and 17% of participants who made child-care and other routine trips, respectively, did so primarily through highway travel. Most frequently, trips were made through a combination of travel on highways and signalized roadways. Given the road-type use trends among partici-pants, and because traveler information is available mostly on highways, approximately a third of participants (those using primarily signalized and neighborhood roads) who made a specific type of trip would likely not have traveler information relevant to their trip. Figure 9.3 displays these results.Participants unanimously indicated that for every type of trip they made, arriving on time was between âsomewhat importantâ and âvery important,â based on a 5-point scale, with 3 equaling âsomewhat importantâ and 5 equaling âvery important.â All 36 experiment participants (100%) who indicated they made school trips identified arriving on time as very important. Nearly all (86% and 88%, respectively) experiment participants who indicated they made trips to Pre-Experiment Survey, Sample StatisticsThe participantsâ median age range was 40 to 49 years. Nearly half had a college degree or higher, whereas only 25% of the U.S. population (age 25 and older) has completed a college or postgraduate degree. While most participants made work trips (94%) or trips for a medical appointment (94%), a few participants did indicate that they never made these types of trips (6%). Conversely, 55% and 60% of participants never made school-related or child-care trips, respectively. In addi-tion, although nearly all participants made work-related trips, only 60% commuted daily to work. Furthermore, only 13% commuted daily to either school or child care. As expected, no participant made daily medical-related trips. Figures summarizing basic statistics related to participantsâ gender, age, education, and types of trips made are included in Appendix G.Approximately 25% of participants that made trips to work, school, and medical appointments traveled using mostly Table 9.6. Average Threshold for Late Arrival, by Importance of On-Time Arrival and Trip Purpose (min)Arrival ImportanceTrip Destination by PurposeAll TripsWork School Child Care Medical Other1 na 4.8 20.0 na 15.0 9.02 na 10.0 25.0 5.0 22.5 17.03 17.0 6.0 7.0 7.1 9.0 9.24 5.1 5.6 11.8 7.3 10.7 8.35 4.9 6.0 3.3 5.7 5.5 5.1Average lateness threshold 5.8 5.9 6.0 6.2 9.3 6.7Note: na = not applicable. in the first column, 1 = unimportant and 5 = very important.Table 9.7. Willingness to Pay for Trip Reliability and Information on Late Arrival, by Importance of On-Time Arrival and Trip PurposeArrival Importance (1 = unimportant, 5 = very important)Eliminate Trip Variability (Guaranteed Travel Time)Know Youâre Going to Be Late (Late Arrival Traveler Information)Work TripsSchool TripsChild-Care TripsMedical TripsOther TripsWork TripsSchool TripsChild-Care TripsMedical TripsOther Trips1 na na na na na na na na na na2 na na na na na na na na na na3 $0.55 na na $0.78 $0.30 $0.30 na na $0.58 $0.254 $0.52 $0.75 na $0.23 $0.34 $0.59 $0.54 na $0.45 $0.455 $1.38 $1.18 $1.17 $0.98 $0.73 $1.23 $0.91 $0.86 $0.90 $0.14Average WTP $1.20 $0.93 $1.05 $0.77 $0.37 $1.08 $0.73 $0.73 $0.74 $0.28Note: WTP = willingness to pay. na = not applicable, because there were fewer than five observations (fewer than five people were willing to pay for the information).
94Figure 9.3. Types of roads participants use for specific trip types.Figure 9.4. Participantsâ rating of importance of on-time arrival, by trip purpose.participants who made child-care trips experienced very low levels of trip variability (0 min to 10 min of extra travel time), while only 41% making work trips had the same low level of trip variability. One in four participants who made work trips indicated that on a bad day, their trip can take 30 or more additional minutes beyond their average trip time; only one participant had that level of variability for his or her child-care trip.Yet, participants defined late far more precisely for work and child-care trips compared with other trip types. One in five participants who made work trips categorized arriving late as arriving even 1 min beyond his or her scheduled arrival time, and another one in five set the late-arrival bar at 2 min within the scheduled arrival time. Late arrivals for child-care trips were perceived by participants as even more time sensi-tive. These outcomes are presented in Figure 9.7.work or medical appointments also identified arriving on time as very important. Figure 9.4 presents a chart summa-rizing participantsâ ratings for on-time arrival importance by trip type.Figure 9.5 presents a chart summarizing participantsâ normal trip time for the subset of participants that made a specific trip type. Most participantsâ (58%) average work-trip time ranged between 10 min and 30 min, while 30% of participants who traveled to work experienced an average travel time greater than 30 min. Participantsâ medical appointments appeared to have a similar average trip time distribution to participantsâ work trips. Average travel times for work and medical-related trips were generally greater than average travel times for school and child-care trips.Figure 9.6 presents a chart summarizing participantsâ additional travel time on a bad day. More than 50% of
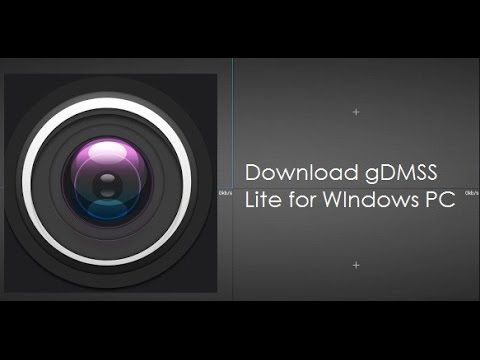
95 Figure 9.7. Various definitions of late arrival, by trip type.Figure 9.5. Average trip duration, by trip type.Figure 9.6. Trip time variability, by trip type.
96Figure 9.8 presents a chart summarizing participantsâ will-ingness to pay for a guaranteed travel time to their destina-tion. More than 80% of participants were willing to pay something to eliminate the variability in travel to work, but only 42% of participants who made other routine trips were willing to pay money to reduce variability on those trips. Such trips have a far lower on-time arrival importance. On average, participants who made work trips were willing to pay $1.20 per trip to eliminate trip variability. For child-care trips, the value was $1.05; and for school-related trips, the value was $0.93.Surprisingly, nearly as many people (76%) were willing to pay for information showing that they would arrive late for a work trip as were willing to pay to reduce their work-trip time variability (81%). Furthermore, they were willing to pay nearly as much for the information ($1.08 versus $1.20), even though the information would not improve their travel time or trip outcome. The average willingness-to-pay amount for school, child-care, and medical trips was the same, at $0.73 per trip. Figure 9.9 presents a chart summarizing participantsâ willing-ness to pay for late-arrival knowledge.Post-Experiment SurveyParticipants were asked four brief questions after complet-ing the simulation experiment. The second question asked how they perceived the usefulness of traveler information at the start of the simulation and after completing the experi-ment. Figure 9.10 and Figure 9.11 present participantsâ responses.At the beginning of the experiment, about half of the participants in the L1 and L2 groups were neutral (48% and 52%, respectively) about the usefulness of the traveler information; fewer believed it was somewhat useful (44% Figure 9.8. Willingness to pay for guaranteed travel time, by trip type.Figure 9.9. Willingness to pay for late-arrival knowledge, by trip type.
97 and 30%, respectively). Many more L3 participants rated the information as somewhat to very useful (57%) than rated it neutrally (33%). At the end of the experiment, the L1 and L2 groups who were neutral shifted signifi-cantly more toward the positive and slightly toward the negative. The L3 group had a far less pronounced shift to the positive.During the post-experiment survey, participants also revealed how frequently they sought out traffic information for the work trip and the types of information they used to acquire this information. Participantsâ responses were aggre-gated and are presented in Figure 9.12 and Figure 9.13. Most participants frequently sought out traffic information, and 30% did so every day. Most used multiple media to acquire the information. More than half of the participants who sought out traffic information for the work commute used the radio.experimental resultsParticipants who received all three information sets (qualita-tive messages on roadside message signs along route, real-time travel time at 5-min granularity, and static travel time ranges based on time of departure) were referred to as receiv-ing L3 information. Participants who received the qualitative messages and real-time travel time information were referred to as receiving L2 information. And participants who received only the qualitative messages were referred to as receiving L1 information.Participants with L3 information were more conservative in their departure time decisions than the L1 and L2 groups on non-event days. This result likely occurred because the reliability information that supplemented L3 participantsâ real-time information increased their awareness of trip vari-ability and led them to budget more travel time. Only 23% of Figure 9.10. Ratings of traveler information usefulness at beginning of experiment.Figure 9.11. Ratings of traveler information usefulness at end of experiment.
98participants with reliability information departed at 8:15 a.m. on non-event days, while 30% and 45% of participants receiving L1 and L2 information, respectively, departed at 8:15 a.m. Conversely, L2 participants budgeted the least travel time for their trips. Table 9.8. summarizes participantsâ departure time decisions by information level for event and non-event days.The trip objective for participants was to depart no earlier than 8:00 a.m. so they could spend that time checking in on family. At the same time, participants were asked to arrive by 9:00 a.m. to work to avoid a $25 late-arrival work penalty but not much earlier to avoid parking fees of $4.00 per hour. Note that all of the motivations constructed for these simulated trips were hypothetical, and no variations in participant compensation were tied to performance within the experi-ment. Given that L3 participants chose to depart earlier than L1 and L2 participants, they more often missed time with their family. L3 participants missed morning family time on average 2.6 out of 10 days, while L2 participants missed on average 2.0 out of 10 days.Participants with reliability information (L3) arrived late 1.5 days out of 10 on average, while their counterparts with L2 and L1 information arrived late on average 2.1 days out of 10. The average total late-arrival cost for participantsâbased on the experimental framework and 10 commute daysâwas $45, $39, and $28 for L1, L2, and L3 participants, respectively. The average early arrival costs were $11, $9, and $11 for L1, L2, and L3 participants, respectively. Table 9.9 breaks down late and early costs by week and by event and non-event days. Based on the cost layouts presented to experiment participants, those receiving reliability information in addition to the other sets of information (L3 participants) had the overall lowest sched-ule offset cost of $38.61. In-vehicle travel time costs are not a component of the calculations presented in Table 9.9.Figure 9.12. Frequency of seeking traffic information for work trips.Figure 9.13. Media used in acquiring traffic information for work trips.
99 Table 9.8. Aggregate Departure Decisions for Groups with Different Traveler Information Departure TimeNon-Event Days Event DaysL1 (DMS)L2 (DMS 1 RT)L3 (DMS 1 RT 1 REL)L1 (DMS)L2 (DMS 1 RT)L3 (DMS 1 RT 1 REL)7:45 16% 7% 17% 30% 34% 34%8:00 54% 48% 60% 51% 40% 48%8:15 30% 45% 23% 19% 26% 18%Note: DMS = dynamic message sign; RT = real-time travel time; and REL = reliability information.Confidence in On-Time Arrival Was Tempered with Reliability InformationOn average, participantsâ level of confidence in an on-time arrival at the beginning of each trip was equivalent among the three traveler information groups and did not vary significantly from week 1 to week 2. At Waypoint 1 (first decision point), for non-event days, arrival confidence on average was nearly the same as at the beginning of the trip. However, on event days, participantsâ levels of confidence in on-time arrival decreased by 8%, 21%, and 13% for L1, L2, and L3 participants, respec-tively. Having quantitative information (L2) on delay caused a significant decrease (4.2 to 3.3, average from trip start to Waypoint 1) in level of confidence. Knowing overall variabil-ity of the trip in addition to having quantitative information (L3) mitigated this degradation in confidence (4.1 to 3.5, average trip start to Waypoint 1).At Waypoint 2 (second decision point), the trend was sim-ilar to Waypoint 1 in that arrival confidence was lower for event days compared with non-event days. L1 participants continued to have less confidence in an on-time arrival from Waypoint 1 to Waypoint 2. L2 participantsâ average level of confidence increased from Waypoint 1 to Waypoint 2; at Waypoint 2, they often passed the event causing delay and had additional information increasing their confidence in an on-time arrival. The average level of confidence for L3 par-ticipants was equivalent from Waypoint 1 to Waypoint 2. Fig-ure 9.14 presents graphically the average change in level of confidence in an on-time arrival from the beginning of the trip to Waypoints 1 and 2.Reliability Information Reduced Pre-Trip and En Route Stress for Unfamiliar TripsThe level of serenity along trips was solicited through the questions asking about levels of stress at specific points along the trip. At the first waypoint, participants generally selected values representing neutral (value of 3) to stress-free travel (value of 5). Higher levels of traveler information generally did not equate to less stress at the first trip waypoint. Interest-ingly, for participants receiving L1 information, the first com-mute day was the day with the highest average level of stress at the first waypoint, followed by the eighth commute day (bad weather and highest travel times). For L2 participants, the average stress level at Waypoint 1 was nearly equivalent Table 9.9. Schedule Offset Costs Based on Experiment FrameworkCosts for Schedule Offset Based on Experimental FrameworkLate Arrival Cost Early Arrival Cost Total Cost*L1 L2 L3 L1 L2 L3 L1 L2 L3All 10 Simulation Days $45.19 $38.54 $27.50 $10.36 $8.88 $11.11 $55.55 $47.42 $38.61Week one $18.27 $20.83 $15.83 $5.52 $4.05 $5.46 $23.79 $24.89 $21.29Week Two $26.92 $17.71 $11.67 $4.84 $4.82 $5.65 $31.76 $22.53 $17.32Non-Event Days $10.58 $9.38 $5.00 $6.40 $4.86 $6.77 $16.98 $14.24 $11.77Event Days $34.62 $29.17 $22.50 $3.95 $4.01 $4.34 $38.57 $33.18 $26.84* Total cost includes only early and late schedule offset and not in-vehicle travel time.
100for the first commute day and event day 6. On most event days, L3 participants found travel more stressful at Waypoint 1 compared with the first commute day. The higher serenity levels for the first commute day (at the beginning of the trip) for L3 participants coupled with their higher willingness to pay for traveler information at this waypoint suggested that reliability information for unfamiliar trips has value.The level of serenity (1 = very stressful, 5 = stress free) selected by participants at the end of the trip was nearly equiva-lent to that at the first waypoint across all non-event days (aver-age rating of 4.1). Further inspections revealed that the level of serenity (stress reduction) was lower (by 0.4â0.5 points) for non-event days in the first week compared with non-event days in the second week. This finding again illustrates the learning curve with unfamiliar trips. Furthermore, as expected, participantsâ level of serenity for event days was on average 20% lower than on non-event days (4.1 versus 3.2). Average differences among groups with different information levels did not prove significant for end-of-trip serenity levels. The question about happiness associated with trip outcome yielded findings that were nearly identical to the question about level of stress information at trip end.Willingness to Pay for Traveler Information at Beginning of Trip Reflected Value of Reliability InformationThe possible range of willingness to pay that participants could select at the beginning of the trip was $0.00 to $1.50. At the beginning of each trip day, participantsâ willingness to pay for traveler information was slightly higher on average for L3 information ($0.61) compared with the other two groups ($0.54 for L1, $0.55 for L2). Furthermore, the willingness to pay trend line had a negative slope for L3 participants. Pro-viding reliability bands, in addition to real-time travel times, helped participants become familiar with the work trip more quickly; consequently, their willing to pay for information at the beginning of the trip diminished further into the exper-iment. Conversely, the willingness to pay trend line had a positive slope for L2 participants, suggesting that the real-time information was continuing to help them gain an inherent understanding of the tripâs variability. Figure 9.15 presents these trends; however, these trends likely would have changed had participants completed additional simu-lation days.The possible range of willingness to pay from which partici-pants could select at the end of the trip was $0.00 to $5.00. The average among the three groups was $2.30. Surprisingly, this measure did not show significant sensitivity in aggregate from week 1 to week 2 or for event and non-event days. Participants were, on average, willing to pay only slightly more ($0.02 to $0.07) for traveler information on event days compared with non-event days; however, these differences were not statisti-cally significant. Further, participants receiving L1 infor-mation were, on average, willing to pay $0.15 to $0.17 more per trip compared with participants receiving L2 and L3 information.Traveler Information Provided Trip Serenity Even When Trip Outcome Could Not Be ImprovedTraveler information can benefit travelers even when the infor-mation itself does not reduce travel time by allowing Figure 9.14. Average on-time arrival confidence during trips on event days.
101 travelers to adjust their schedule to accommodate a late arrival. In the simulation experiment, participants were allowed an opportunity to simulate a call to work to inform their employer that they would be arriving late (and avoid the $25 late-arrival penalty). This penalty avoidance could be used only once during the simulated 2-week period. Researchers compared the trip characteristics and trip experience for each participant who used this opportunity against another trip made by the same individual in which the outcome was also a late arrival but the call option was not exercised. The expecta-tion was that having the opportunity to call the employer would provide a level of serenity that would translate into lower trip stress, greater happiness with trip outcome, and higher perceived usefulness of traveler information compared to a comparable trip without the call option.Among the 80 participants, 33 arrived late and chose to inform their employer of a late arrival. Of those 33, 11 called their employer at the first waypoint, and 22 did so at the sec-ond waypoint. Of the 33 participants, 7 did not arrive late any other day. Consequently, the sample set became 26 because a point for comparison was not available for those 7 partici-pants. A few of the 26 participants arrived late on multiple days. For this circumstance, the day with an arrival delay simi-lar to the day they chose to call their employer was selected for a paired comparison.Of the 26 paired trips analyzed, 6 pairs were made by par-ticipants who received L1 traveler information, 11 pairs were made by participants who received L2 traveler information, and the remaining 8 were made by participants who received L3 traveler information. Twenty of the 26 paired trips had the same departure time. In three pairs, the trip during which the call to employer was made began at 8:00 a.m., while its counterpart began at 8:15 a.m. The reverse occurred for the remaining three pairs.On average, the 26 trips for which a call for late arrival was made required 5 min of additional in-vehicle time and ended on arrival 5 min earlier than their paired counterparts. When able to call their employer regarding a late arrival, partici-pants found the trip less stressful, were happier with their trip outcome, and found traveler information more useful. The difference with regard to happiness with trip outcome was statistically significant at the 99% level, and the differ-ence in the usefulness of traveler information was statisti-cally significant at the 95% level. All other differences were statistically significant at the 90% level with the exception of willingness to pay, where the difference was significant at the 80% level. Outcomes of the paired analyses are presented in Table 9.10.The difference in travel times and arrival delay were statis-tically significant between the paired sets, which suggested that the trips may not actually have been equivalent with regard to travel times and arrival outcome. On further inves-tigation, researchers observed that, for 14 of the 26 paired trips, the difference in arrival delay was greater than 10 min and that those differences may have clouded the assessment of serenity benefits. Therefore, a second analysis with only 12 paired trips was conducted.The more focused analysis confirmed that knowledge of a late arrival en route provided a serenity benefit as captured by lower trip stress, greater happiness with trip outcome, and higher rating for traveler information usefulness. In this analy-sis, the difference among pairs was not statistically significant for in-vehicle travel time and average minutes late. Further-more, for this sample, the average difference in willingness to Figure 9.15. Trend in willingness to pay (at beginning of trip) across simulation days.
102by week and by event and non-event days. As expected, total per-participant trip costs on event days were far greater than costs on non-event days, and the differences were statistically significant. Difference in cost among different traveler infor-mation groups was not statistically significant for event days.The schedule offset costs as outlined in the experiment and the trip costs based on Small et al.âs model admittedly are inherently different in form; consequently, they cannot be directly compared. Small et al.âs model has a greater penalty for very early arrivals and lesser penalty for late arrivals com-pared with the simplified framework of this experiment. Fur-thermore, the total costs based on the experimental framework do not include in-vehicle travel time.What does prove useful from this experiment is participantsâ valuation of trip serenity. Given participantsâ stated willingness to pay for traveler informationâand differences in this value when participants could simulate a call to their employer while having the same trip outcomeâthe research team sug-gests adding a term to the Small et al. model to include a cost discount for the serenity benefit of traveler information. The proposed model is this:2 22c T SDE SDE SDL D DSDE SDE L I( ) ( ) ( )= α +β + β + γ +θ + λpay for traveler information was $0.25 and was statistically significant. These findings are presented in Table 9.11.application of results in travel Utility FunctionsA typical method for valuation of trips to work involves the application of the cost function (utility model) proposed by Small et al. (1999). This model, based on a population of commuters along SR-91 in California, estimates that in-vehicle travel time costs $3.38 per hour, which the authors professed is low. They attributed the low value to partici-pants placing greater interest on trip variability trade-offs. The cost per hour for late arrival is $18.60, with a $2.87 one-step penalty for arriving late. Furthermore, the modelâs cost for early arrivals provides a quadratic penalty for very early arrivals.Application of this utility model, and model parameters, to all trips simulated in this experiment yielded an average cost of $5.25 per trip, or $52.55 for the set of 10 trips. This cost includes early- and late-arrival costs as well as in-vehicle travel time. Trip costs were slightly lower for L3 participants compared with L1 and L2, but the differences were not statis-tically significant. Table 9.12 breaks down late and early costs Table 9.10. Value of Late-Arrival Information, Expanded Sample SizeTrip Metrics Sample Size 5 26 Paired TripsAverage Trip ValueStatistical Sig. of Differences in Paired DataParticipants Phoned AheadPaired CounterpartAverage in-vehicle travel time (minutes) 61.2 56.3 95%Average minutes late 13.9 18.8 95%Trip Stressfulness (1 = very stressful, 5 = stress free) 1.92 1.81 75%Happiness with trip outcome (1 = unhappy, 5 = very happy) 2.12 1.31 99%Usefulness of traveler information (1 = not useful, 5 = very useful) 3.88 3.19 99%Willingness to pay for traveler information ($0â$5) $2.73 $2.50 85%Table 9.11. Value of Late-Arrival Information, Specific Paired ComparisonTrip Metrics Sample Size 5 12 Paired TripsAverage Trip ValueStatistical Sig. of Differences in Paired DataParticipants Phoned AheadPaired CounterpartAverage in-vehicle travel time (minutes) 58.2 56.4 75%Average minutes late 8.2 7.7 75%Trip Stressfulness (1 = very stressful, 5 = stress free) 2.33 1.75 93%Happiness with trip outcome (1 = unhappy, 5 = very happy) 2.50 1.50 99%Usefulness of traveler information (1 = not useful, 5 = very useful) 4.25 3.25 99%Willingness to pay for traveler information ($0â$5) $2.75 $2.50 96%
103 The first hypothesis stated that provision of accurate reliability information would result in improved on-time performance and lower generalized travel disutility com-pared with a control group receiving no reliability informa-tion. Findings from the study supported this hypothesis. Participants who received reliability information were on time more frequently (85% versus 79%) and had lower total late- and early-arrival penalties associated with the simulated trip ($38.61 versus $47.42 and $55.55 in the two control groups). Both findings were statistically significant with 95% confidence despite the relatively small sample size of the experiment.The second hypothesis proposed that, while travel out-comes would improve with the provision of reliability infor-mation, the perceived value of the reliability information would underestimate the realized benefit in terms of reduced delay, improved on-time reliability, and reduced stress. Findings from the study supported this hypothesis as well. Measured perceptions of benefit associated with reliability information were not commensurate with the observed improvements in trip outcomes. Changes in the value of information and stress reduction, although generally favor-able to reliability information, were not statistically signifi-cant. The implication is that although reliability information can be useful in managing trip time variability, the apparent value of the information is lost in the context of learning over time. The experimentâs subjects likely internalized the reli-ability information as one element in the learning process, rather than considering its value outside of the learning pro-cess. Providers of reliability information may face an uphill battle in measuring perceptions of the effects of reliability information even when such information is useful in improv-ing trip outcomes.The third hypothesis was that the benefits of reliability information would decline over time as both experimental and control subjects learned and internalized an understand-ing of underlying travel time variability. That is, the benefit from reliability information in the first few weeks would be larger than in the last few weeks. Findings from the study were where c = cost of travel (disutility) T = travel time SDE = schedule delay early SDL = schedule delay late DL = late-arrival index = 1 if 00 otherwiseSDL > DI = late-arrival information index = 1 if late-arrivalinformationis acquireden route0 otherwise Based on this model form, the l reflects the reduction in trip cost from having information en route that the outcome of the trip will be a late arrival. The proposed value l accord-ing to the findings of this study, would be -$0.25. This value is based on an average trip length of 55 min, with an approxi-mate travel time range of 45 min to 70 min. On average, partici-pants received traveler information of late arrival 35 min into the trip, which was 20 min before their trip end and 15 min before their planned arrival.Note that, in the pre-experiment survey, participantsâ aver-age willingness to pay for knowledge of a late arrival for the trip to work ranged from $0.30 to $1.23, depending on how important on-time arrival was for the group. Overall, average willingness to pay was $1.08 based on a 10-point scale from $0.00 to $5.00. Compared with the findings from the experi-ment, these findings illustrate that stated willingness to pay was somewhat higher than what was measured in the simula-tion; but both the stated and the observed significance of information on late arrivals was valuable.Conclusions and Next StepsThis section summarizes experimental findings with respect to the three key experimental hypotheses. In addition, a number of next steps are identified for possible inclusion in possible follow-up experimentation.Table 9.12. Application of Small et al. Utility FrameworkCosts for Schedule Offset Based on Small et al. FrameworkLate Arrival Cost Early Arrival Cost Total Cost*L1 L2 L3 L1 L2 L3 L1 L2 L3All 10 Simulation Days $10.84 $11.87 $9.69 $24.08 $18.52 $25.02 $53.76 $50.62 $53.05Week one $5.69 $7.91 $6.37 $14.05 $9.10 $13.06 $28.62 $27.19 $28.40Week Two $5.14 $3.96 $3.32 $10.03 $9.42 $11.97 $25.14 $23.43 $24.65Non-Event Days $1.89 $1.48 $0.82 $15.64 $10.35 $16.31 $24.11 $19.80 $23.43Event Days $8.95 $10.39 $8.86 $8.44 $8.16 $8.71 $29.65 $30.83 $29.61* Total cost includes late and early schedule offset plus in-vehicle travel time.
Idmss Lite Free Download For Windows Pc
104a positive overall usefulness of traveler information at the end of the experiment than at the beginning. In contrast, in the group with reliability information, the swing toward the posi-tive proved more muted. This finding suggests that as the groups without reliability information used the real-time information to learn about trip variability, more individuals found value in the information. For those with reliability information, trip variability knowledge was already present; consequently, its usefulness remained consistent from the beginning to the end of the experiment. Overall, however, a 2-week period was observed to be too short to make any definitive statements regarding this hypothesis.inconclusive on this point. Participants with access to reliabil-ity information did see total early- and late-arrival penalties decline from week 1 to week 2 of the simulated trip-making. Control groups who did not receive this information were mixed, with one group realizing a reduction in total costs and another seeing a rise in those costs. Interestingly, participantsâ willingness to pay for reliability information declined over time in the simulation experiment, indicating that experience within the simulated trip began to offset the reliance on pro-vided reliability information.According to the post-experiment survey, for groups with-out reliability information, many more individuals perceived
Next: Chapter 10 - Enhanced Laboratory Experiment »Narrow Results
By Price
By Category
By Operating System
Faux Fur Laptop Case
- Web sitehttp://www.dahuatech.com
- Support web sitehttp://www.dahuatech.com
Download Gdmss For Laptop
- Support e-mail Not provided
- Support phone Not provided
Download Dmss Fur Laptop Download
- iPhone surveillance software iDMSS Lite version, which support view and control live video streams from cameras and video encoders.26,50278
- iPhone Surveillance Software iDMss Plus, which support Push Alarm, Video Playback, Task wheel, Finger Gesture, Windows Slide and so on.8,66360
- iPAD Surveillance Software iDMSS HD Lite vision, which support view and control live video streams from cameras and video encoders.2,1357
- iPad Surveillance Software iDMss HD, which support Push Alarm, Video Playback, Task Wheel, Finger Gesture, Windows Slide and so on.4514
- DSS Mobile is mobile surveillance software designed for security field, working together with Dahua DSS platform. It has user-friendly UI and...1702
- 1.NVR2.3.712
- The AMS application enables remote management of the alarm system based on the Dahua control panels. It allows you to easily monitor and control...1241
- VTMC is an APP offering free audio/video calls for Video Intercom. It is suitable for devices that using SIP protocol and can be used in villa and...181
- VideoView Mobile HD for iOS, which supports live viewing, playback, push alarm, and other advanced options.451
- iPhoneDSS7016 Gisios7ios81,4101
- Results 1 - 10 of 13